By EloInsights
- Technology is constituted and makes sense through people. For this reason, it is also capable of carrying biases and reproducing discrimination that affects everyone, including companies.
- Emerging inventions, such as artificial intelligence, already support and make various kinds of decisions. The danger lies in being guided by a false neutrality of technology, ignoring its social dimension.
- Through practical examples, we explain how algorithmic racism affects people. Fighting it back involves building a corporate culture that respects and promotes diversity.
The use of facial recognition software may seem trivial to a white person. The technology is already embedded in various devices, like our own smartphones, and used in everyday activities, such as validating access to airports, schools, companies and even in the electronic gates of residential buildings. However, for a black person, or one belonging to other minority groups, this experience can be an aggressive reminder of the biases contained in our social structure, whether it is because their faces are not even detected or because their features can be mistaken for those of other people.
This problem is partly explained by the lack of diversity in the images that feed databases used to train devices based on artificial intelligence (AI). The low variety of phenotypes (skin color, hair textures, eye shape and color, etc.), lead to a path in which this type of technology has a much greater chance of being confused when comparing, for example, the faces of two black people, than it does of mistakenly identifying two white people.
Situations like these are part of what experts call “algorithmic racism”. This phenomenon refers to the unfolding of our unconscious biases in algorithms that order the functioning of devices and end up reproducing oppressions in digital environments. The result is that various emerging technologies are subject to reproducing stereotypes and asymmetries embedded in the collective imagination throughout history.
“I define algorithmic racism as the way in which the current arrangement of technologies, social and technical imaginaries strengthen the racialized ordering of knowledge, resources, space and violence to the detriment of non-white groups”, explains Tarcizio Silva, a researcher associated with the Mozilla Foundation and author of the book Racismo algorítmico: inteligência artificial e discriminação nas redes digitais (Algorithmic racism: Artificial Intelligence and Discrimination in Digital Networks, in a free translation to English).
For Silva, the main problem is not in the lines of code, but in reinforcing and favoring the reproduction of designs of power and oppression that are already in place in the world. The implementation of digital technologies is not exclusively technical in nature and, therefore, cannot be uncritical. It also has social implications, which must be addressed by companies.
Pedro Guilherme Ferreira, Analytics Director at EloGroup, points to the need of understanding the debate’s level of criticality, not just the potential damage to the companies’ reputations: “Racism is a criminal offence and needs to be combated. Racism in algorithms should be no different. Bias in the data is contained in this universe and, ultimately, can cause great damage”.
In this article, we will mainly discuss algorithmic racism, but any kind of bias or discrimination interferes with the relationship between people and technology, two central elements in the digital transformation process that organizations are going through. Especially when the social dimension gains relevance within ESG agendas. By looking at the “S” in the acronym, we can make a direct link between the theme of this article and the construction of an open corporate culture, capable of engaging stakeholders around strategic goals, without neglecting accountability for possible negative effects on society.
“Different points of view are constructed because of different experiences based on our social markers. In places where there are different perspectives, people will look at the same situation through a different lens”, says Gabriel Leví, Diversity and Inclusion (D&I) leader at EloGroup. “The sum of these various views complements each other and forms a vision that is much closer to reality. This is the immense value of diversity when we think about decision-making”.
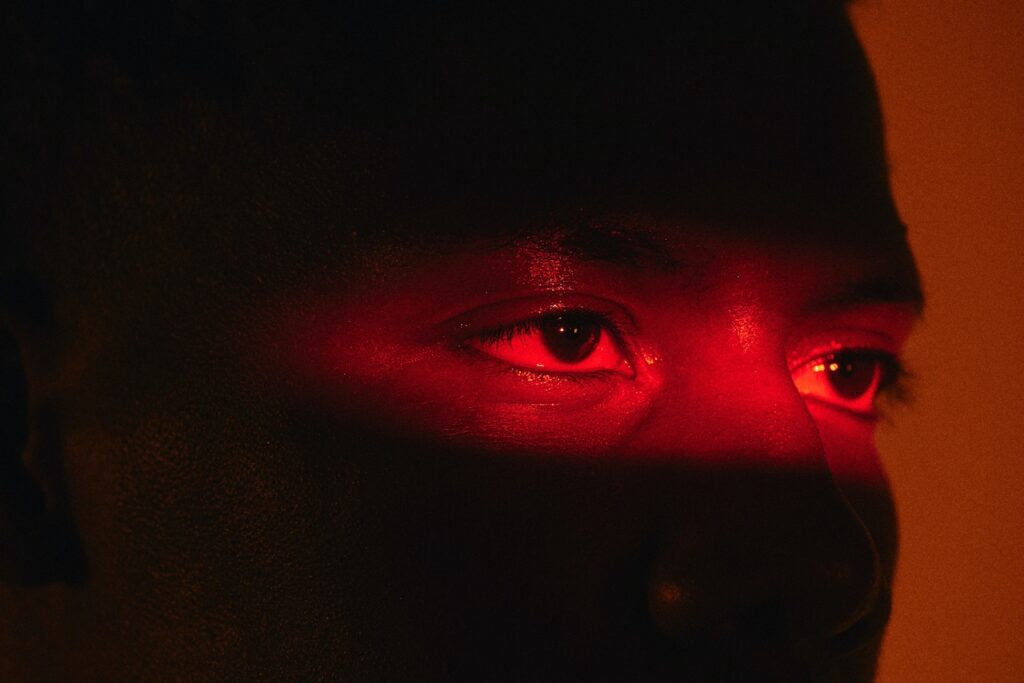
Building a more plural, sustainable and profitable future involves promoting inclusion, equity and diversity in the corporate environment. The generation of value in business is maximized in truly inclusive and diverse environments. Even more so because of the transformative and experimental nature of enabling technologies, such as artificial intelligence and its use in conjunction with algorithm programming.
Furthermore, combating technological bias in organizations is part of caring for a capital that is both human and economic, as it aims to respect people, be they shareholders, employees or customers.
Before we delve into ways of mitigating these biases, let’s understand how it happens.
How and why there is bias in technology
Among many of his works, the specialist Tarcizio Silva keeps a timeline that catalogues how often digital media, social media and AI-based devices reinforce racist bias in society.
The starting point of this collection is 2010, when a feature to prevent selfies with closed eyes, present in Nikon cameras, was confused with the eyes of Asian people. It goes on to other emblematic cases that caused outrage, such as one in 2015, when black people were dehumanized and tagged as “gorillas” by one of Google’s tools. And it goes right up to the present day, in 2022, with complaints such as the iPhone not being able to register the faces of people with traditional Māori tattoos; or a start-up that developed software that modifies different accents to sound like the standard white American; or even the facial recognition system of a banking app that could not identify the face of a black account holder.
As well as being vast, this documentation reinforces that understanding the biases contained in algorithmic systems is not just about analyzing the structure of codes, nor is it about considering such cases as isolated or specific uses. “It involves identifying which behaviors are normalized, which data they accept, which types of error are or are not considered between system inputs and outputs, their potential for transparency or opacity and for which presences or absences the systems are implemented. In short, to analyze the networks of political-racial relations in technology”, states Silva.
Another researcher who is sharpening her gaze on artificial intelligence is Nina da Hora, who defines herself as a scientist in the making, an anti-racist and decolonial hacker. In her view, “the racial bias comes from us, who feed the algorithm. It was not born with the algorithm; it was born with our society. And it is exceedingly difficult to identify at what stage the negative results begin”.
This statement was made in an episode of journalist Malu Gaspar’s podcast. In another extract, she explains how algorithmic racism happens by differentiating between image recognition and facial recognition.
In her words, image recognition often uses algorithms from machine learning trained to recognize important points in the image of a face or an object. From this, there are possible interpretations and decision making. This is the case with social networks, which have pre-collected and organized image bases, such as Facebook, which automatically tags people in a photo.
Facial recognition, on the other hand, usually takes place in real time and is a technology focused on the face. It starts with the eyes and works its way down, looking for expressive marks to identify a person. It also uses algorithm training, in which there is a base of images through which it will make the match, saying whether or not the person corresponds with who is shown in an image collected, for example, by a security camera. Human intervention takes place in both technologies and throughout the collection and analysis process.
For Pedro Guilherme Ferreira, facial recognition tends to be more accurate precisely because it focuses on the geometry of the face. “This technology takes more account of facial features and less of social characteristics. Image recognition is most often done by association via unsupervised learning and can therefore hold more biases, such as racism”, explains the director.
The accuracy of these technologies fails due to multiple factors. The bias can be both in the way the image is captured and in the construction of the logic on which the collected image is analyzed. Without a sufficient variety of faces and phenotypes, a predictive model applied to public safety can misidentify a mark of expression and point to an innocent person as guilty, for example. But not only the image bases used to combat crime make negative associations with the characteristics of minority groups, such as black, Latino, Asian and other non-white identities.
When recognizing images in search engines on digital platforms – which are widely used for services and products, for example –, the hair texture with black features is identified with pejorative content and associated with negative terms, recalls Da Hora. All of these social stigmas contribute to the identification of white people being more efficient, accurate and with a more positive bias.
The documentary Coded Bias, by filmmaker Shalini Katayya, brings other dimensions to the problem, with layers of experimentation. Researcher Joy Buolamwini, PhD and creator of the Coded Gaze and Algorithmic Justice League projects, recounts how an art project using computer vision at the MIT (Massachusetts Institute of Technology) lab made her change direction and study various facial recognition platforms in depth.
Her first idea was to create a mirror that would work on self-esteem and inspiration with the effect of superimposing the faces of ordinary people onto those of personalities, such as tennis player Serena Williams. However, tests with the software used to make the project viable did not work. At this point, the documentary shows scenes similar to those described so far.
When in front of the machine, Buolamwini’s face is simply not recognized. The reading was only possible when, out of curiosity, she decided to put on one of the lab’s costumes: a completely white mask. When she puts it on, the program recognizes her face, but when she takes it off, her real face is not detected. When it analyzed the parameters of the technology, it found that the database was mainly sourced from images of white-skinned people. As a result, the system did not learn to recognize faces like hers, of a black woman.
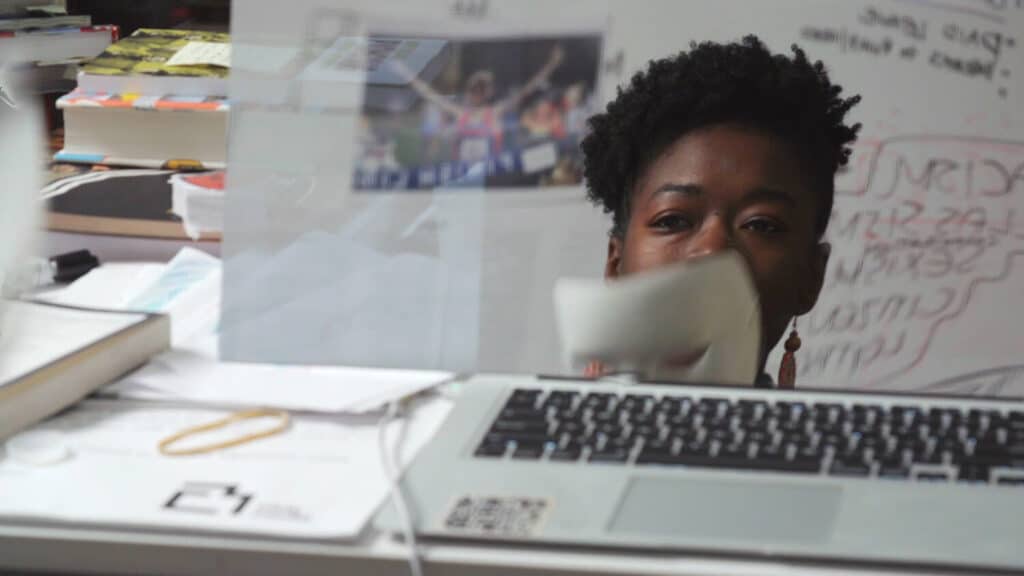
The researcher then decided to extend her investigation to other platforms and found that algorithms from giants like Microsoft, IBM and Google performed better with male faces compared to female faces. They also had better results when detecting faces with lighter skin tones, compared to darker skin tones. The big techs were called in and improved their systems to correct the flaws. The crux of the matter, however, which is the bias in technology, is far from a solution.
“AI is geared towards the future, but it is based on data and that data reflects our history. The past is imprinted in our algorithms”, highlights Buolamwini.
In another passage from Coded Bias, Cathy O’Neal, PhD and author of the book Weapons of Math Distruction, reinforces the concern about how AI could further affect our lives if inaccurate algorithms continue to be inserted into our daily lives. Even loaded with biased readings of the past, they are already used to answer questions such as “will this person pay back this loan?” or “will they be fired from their job?”.
With the participation of many other experts, the documentary points out that machine learning is still not fully understood, and its development is still restricted to a hegemonic and not truly diverse group of people, as it requires a high degree of technical knowledge.
Traditionally, the construction of a code resembles a list of instructions, in which the programmer dictates the rules, and the machine executes. With the evolution of artificial intelligence technologies, coupled with the spread of social media and the massive production of data on the web, machines are now able to learn by interpreting and decoding a mass of information, data sets. So, the algorithm gains autonomy and ends up with a “margin of maneuver” that is beyond the control of the developers. For all these reasons, reflection is urgent and there are many ways to act against biases in technology.
How to overcome algorithmic racism
The world of technology is becoming increasingly inseparable from the social context. Whether it is in everyday tasks like giving voice commands to a device, using filters on social media or, even, in a slightly more veiled way, in systems that determine if you will have access to a university place and bank credit. With this growing influence on all kinds of decision-making, we need to understand how the social sphere is affected.
Algorithmic racism is part of the issue of bias in technology and its solution is as complex as learning how emerging technologies work, especially AI.
Living with diversity and always being vigilant are essential in Pedro Ferreira’s view. “If you have a heterogeneous team, that is already a good start. Also be careful with the data you are using. Flawed data will probably generate flawed algorithms. Finally, it is particularly important to consider the problem of causality by avoiding spurious relationships. New areas within AI, such as causal modelling, are beginning to shed light on solving these problems”, says the director of EloGroup.
Gabriel Leví expands the perspective beyond technical development: “It is also a question of how people can access opportunities to demonstrate their value”. The D&I specialist gives some examples of actions, from the most complex to the simplest:
A cosmetics giant partnered with a school specializing in developers to train its technological staff within a diversity framework. At the end of the nine-month process, some of the professionals were hired. The rest took part in a fair and were placed in technology vacancies at other companies.
Smaller actions in selection processes can revolve around mentoring for people from minority groups. In this case, these employees undergo 2 or 3 months of training before applying for part of the jobs. There is also the possibility of reserving a percentage of vacancies, say 50%, for a specific group of candidates.
Although crucial, the entry point is not the central of attention for companies. It is essential to create the conditions for these people, within their diversity, to be future decision-makers. “This is how they can have an impact, for example, on the logic behind an algorithm. They need to occupy positions of power. Establishing a competency framework helps you understand what the next step is, what skills are needed for that group of people to get there. Besides, you must give people all the support they need to develop”, adds Leví.
The conclusion is that there is no exact step-by-step or list of definitive actions. Often, biases in behavior or judgement are the result of subtle cognitive processes and occur at a level below a person’s conscious perception. This is what Janaína Gama, senior D&I consultant at Mais Diversidade, teaches us. This means that making decisions unconsciously is a human tendency and naturally has an impact on companies.
“We categories reality into our own understanding. We memories the world based on labels and tags”, said Gama at a training session promoted by the EloGroup+ Academy, an initiative of EloGroup’s D&I program. She also emphasized the need to take responsibility and have a firm stance: “You cannot use unconscious bias as a justification for prejudice, racism and homophobia. You must make a commitment to diversity”.
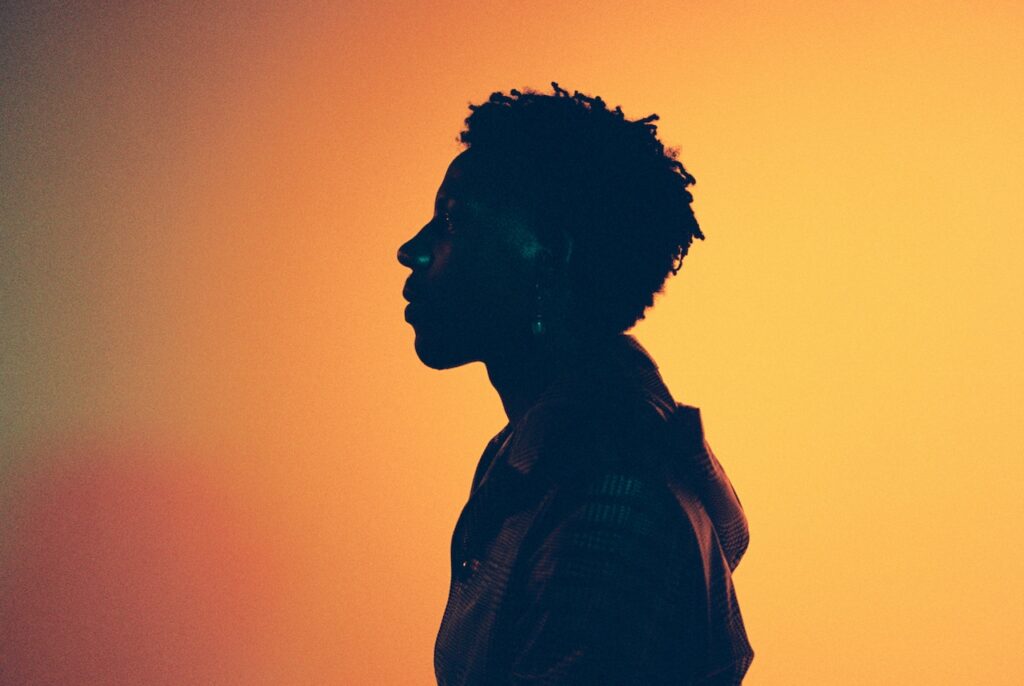
Because of this “subtle” facet, there are still those who deny the existence of racism, which in itself is a huge barrier to overcoming it. However, it does exist, and it is there in the delegitimisation of knowledge and in the blocking of aesthetic and cultural freedoms of social groups.
Tarcizio Silva uses the concept of “microaggressions” to draw attention to the overwhelming amount of aggression that black people face on a daily basis. The term was coined by psychiatrist Chester Pierce between the 1960s and 1970s and refers not to the intensity, but to the high incidence of offences.
“When a black citizen, in her daily interaction with social media and digital systems, has to face problems linked to racism, such as unfair credit scores, differential pricing of services due to her location, or filters that distort selfies, we have constant violence that must be understood and combated”, says the researcher.
In this sense, the fight can be centered on what Silva calls “double opacity”, or the way in which hegemonic groups seek to both present the false idea of neutrality in technology and strive to hinder debate on serious violations, such as racism and white supremacy. “The irresponsible use of supposedly natural databases for training, without full filtering or curation, promotes the worst in society when it comes to data collection. And the layers of opacity in the production of models, implementation and adjustments are defended by large companies, in terms of cost-benefit and business secrecy”, he exemplifies.
Leví uses a phrase from the jurist and philosopher Silvio de Almeida to explain how racism is rooted in society: “’Racism happens when things are normal’. People have the false idea that racism is outside the norm, but it is a status quo. Thinking about specific actions for each type of group is the remarkable thing about diversity. When developing a product, an app or training, you must understand who the people are, what they want to hear and what they have to say”.
At an organizational level, we need to build an open corporate culture, capable of fostering a sense of responsibility in those who develop and use digital assets. Inclusive leadership begins with awareness of our own biases. We need a broad understanding that there are diverse people and that not all social groups are truly included in the market. Affirmative action, in the medium and long term, must be able to bring diversity to the most strategic layers of the organization, influencing more decision-making, diversifying sources of information and promoting moments for everyone to share their stories. This leads to the creation of a corporate environment in which there is psychological security and in which everyone can contribute with high efficiency and creativity.