By EloInsights, in collaboration with Thiago Leite
- We are experiencing a shift towards an autonomous decision-making model in companies, leveraged by data and a large volume of experiments.
- Efforts need to be focused on creating the capacity to experiment, which requires systemic structuring.
- The road to innovation is paved with risk management, data analysis and an agile, disciplined and continuous learning process.
The shift towards a new paradigm in the digital age is shaking up concepts and definitions of innovation and corporate culture. The top-down vision, based on the experiences and intuition of the organization’s top leadership and in which decisions are made according to the hierarchy of positions, is already outdated.
The vision of the future is aligned with an innovative and more grounded horizon, designed by data and leveraged by a continuous process of experimentation. And to make this new decision-making model viable, a large volume of experiments is needed, set up in disciplined rites, so that they solidify in the form of gains that adhere to the organization’s context.
Intuition and professional experience do not lose their relevance, but start to guide the creation of hypotheses that will be confirmed or overturned according to data analysis. In this scenario, experimentation becomes one of the most important skills to develop when it comes to innovation.
It is a learning process with continuous effort and complex measures, but the first steps on this path are less complicated than they might seem. It is true that success with experiments in the digital world involves a series of structures to enable the distribution of decision-making to the ends, unlocking agility and the ability to innovate. Although methodologies and simulations have existed for decades to guide experimentation, the novelty lies in the constant digital transformation that completely changes the dynamics.
One example is the analysis of processes and products in the industrial context. Since the 1950s, the Failure Modes and Effects Analysis (FMEA) method has verified possible failures and their consequences, finding priority improvement actions and hypotheses about the root cause of problems.
Industry 4.0 enhances the analysis, collection and systematization of data using the Internet of Things (IoT) and advanced systems such as: MES, which tracks and documents the transformation of raw materials in industry; ERP, which integrates various departments in business management; and RFID, radio frequency identification that streamlines logistics in supply chains.
Besides that, experiments of various kinds and contexts are being conducted on a massive scale. Discoveries are made on a large scale, at a much faster and more fluid pace. All of this helps to avoid failures and makes the processes leading up to the delivery of products and services more reliable.
In short, paving the way for the autonomy of teams and sectors towards a logic of experimentation requires consolidating databases, connecting systems, performing integrations, digitizing and automating activities to gain speed. Scalability comes as uncertainties are reduced and results are obtained. One way to get a foothold on this learning path is by carrying out the most relevant experiments, those that provide answers on critical elements of the operation, and which can even be automated.
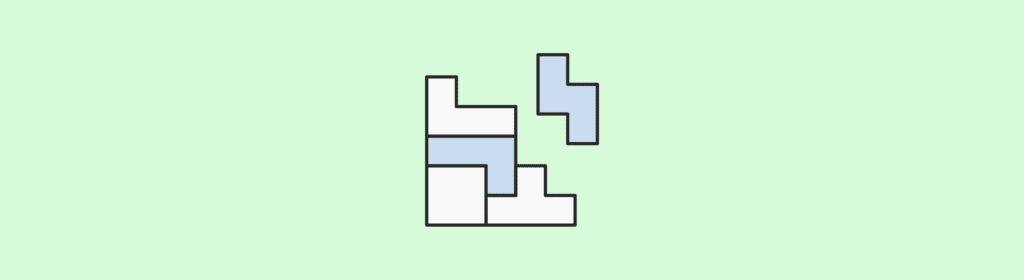
Discipline and developing capability
In practice, the process of experimentation requires method and discipline. It also involves setting up control groups for effective comparisons, analyzing whether the result should be replicated and, in the end, creating the conditions to assimilate learning.
Repetition brings maturity and speed to complete short cycles. The gain in value increases as teams dedicate themselves to improving executions, performing a large volume of tests to reach successful conclusions that point in a constructive direction.
Let us look at experiments related to e-commerce sites. One hypothesis is that changes to the layout of the website or application influence the experience and therefore the customer’s propensity to buy. Based on this, it is possible to test several scenarios: the best place to position the buttons; the best performing algorithm for product recommendations; the level of detail in the product photos. In this case, one of the most widespread tools for A/B testing is Optimezely.
Although these experiments may be considered simpler, they are also more dynamic and have the potential to generate value, given the volume of information collected – at all times, in real time and on the scale that the digital world provides.
It is possible to extend the analysis to hypotheses related to the sales force: what type of ad generates the greatest response; which commercial approach has the best results; how to reduce churn; how to minimize the cost of customer acquisition (CAC), among others.
When developing new products, services and business models that require immersion to understand needs and desires, hypotheses can start from an MVP [Minimally Viable Product]. Getting to a pilot is a way of starting gradually and with minimized risks, starting from a previously defined solution.
It is always important to be careful about the connection between pilot and experiment, because they are not synonymous. The logic of experimentation can start well before a prototype. Conducting a project in an experimental logic means testing a set of hypotheses that will help to build, to understand what a given solution is.
In a more digital reality, other aspects are made easier. Organizations are less dependent on direct interaction – such as focus groups and semi-structured interviews – to understand their customers. With greater access to data, be it studies, statistics or market analysis, face-to-face techniques are still important for developing theories, but they become complementary. It is possible to base business experiments on demographic and behavioral data – often available openly and for free, on many platforms or collected through the organization’s own channels.
A second crucial point is how to carry out the digital transformation, which requires unlocking the company’s potential by establishing a capability-first model. The main thing is to build experimentation skills in the company so that different teams can effectively run and finish test cycles, perpetuating the process.
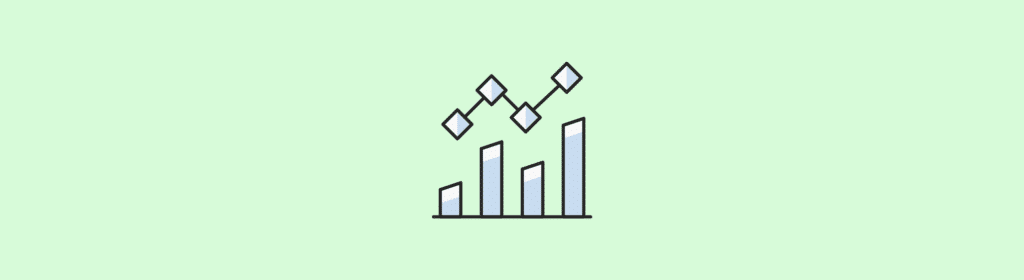
Generating value and balancing risks
In his book Experimentation Works: the surprising power of business experiments, Stefan Thomke makes a correlation between the number of tests within organizations and performance over time on the stock market. In one of his analyses, he shows how among the 500 most relevant companies in the world, part of the S&P 500 index, those that carry out the most experiments are not only considered the most innovative, but also the most coveted by the market.
Of course, multiple factors influence stock fluctuations. The degree to which the company is digitalized – since digital natives tend to stand out – or how well it rides the wave of social media, to name but a few. The fact is that organizations such as Microsoft, Amazon and Booking.com have found that the “everything is a test” mentality generates surprising results and competitiveness, even affecting on share price rises. It is a good indication that experimentation generates value.
Another statistic helps to understand the mechanics of experimentation and the uncertainties involved: only 10% to 20% of experiments end up with positive results. Therefore, if the success rate is much higher than this, it is possible that the company is limiting itself to confirming obvious hypotheses without exploiting their full potential. Similarly, if the rate is too low, there may be an exaggeration in the number of possibilities being considered, which will bring down both conversions and the feeling of confidence. This raises a question: how can we balance the risks and define which hypotheses will be run?
The first step is to link this to the discussion about the operating model. Distributing decision making to the top naturally makes everyone decide according to their common sense or their vision at the time – which entails a huge risk. At the same time, if the decision-making process “wanders” down the organization chart until it reaches a person with sufficient context and information to provide good direction, the company is not moving in real time. Giving autonomy is complex and delegating is not enough to be agile. So, we come back to the need to tie decision making together.
In general, decision-makers carry the risk aversion they feel as individuals into the company, which can hinder the progress of projects. Avoiding isolated tests and easing the burden of each action alleviates this conflict. The risk and return evaluated over a portfolio of experiments tends to increase the willingness to carry out tests and pulverizes potential losses.
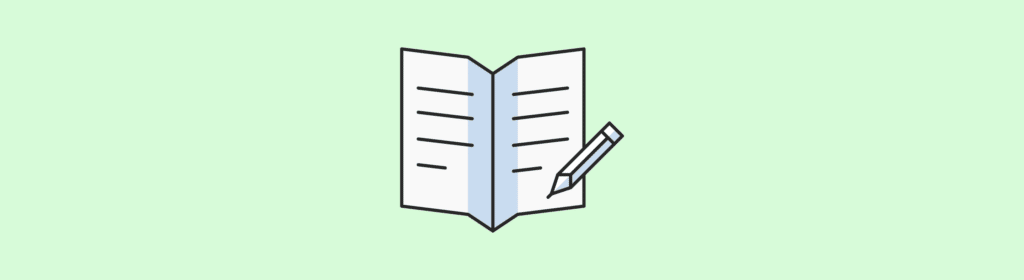
Costs, failures and lessons learned
A change of mindset forces us to face the new, and the resource commitment curve is key to mitigating uncertainties. We have already discussed that by systematizing input data, orchestrating the process of identifying new hypotheses and disciplining the experimentation process, we better balance risks and doubts.
This can mean a significant reduction in costs and even an increase in revenue, depending on the business situation. However, it is a fact that failures will happen – but making mistakes is only a problem when it’s costly. So, it makes a lot of sense to think about the cheapest way to check that something works.
It is the learn to burn concept. The focus is initially on reducing risks before distributing large resources. It branches out into two other imperatives: fail fast, which means fail quickly, and fail smart – a term that is more in line with the issue of experimentation, since it refers to extracting the maximum learning from tests at the lowest possible cost.
Thomke’s work recounts the case of the semiconductor industry, where, for a long time, it was a challenge to obtain detailed data on the performance of equipment and integrated circuits. As a result, engineers had to work out the safety margin in relation to the amount of soldering, for example, or even analyze circuit failures to ensure that the devices could be manufactured.
By collecting data in a structured way and developing sophisticated statistical models in relation to manufacturing capacity, with the incorporation of model design and simulation tools – such as Matlab – it was possible to have a significant impact on safety. Upstream simulation tests increased performance and reduced costs by between 5% and 10%, without reducing manufacturing yield.
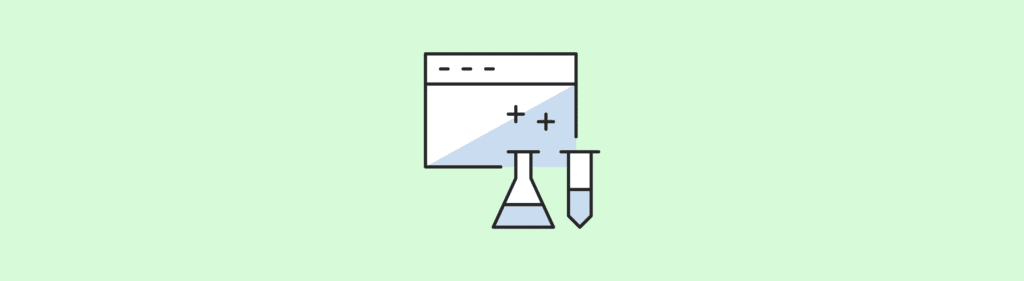
The culture of experimentation
A model that is everything discussed so far is that of Booking.com, which has become one of the largest accommodation search platforms and conducts more than 25 thousand tests a year to reduce uncertainty and improve the customer experience and internal processes. Anyone can test hypotheses without needing the approval of company leaders.
It is a strong example of the practice of test and learn. In this context, as well as being better prepared to deal with surprises, the organization is shaped by transforming frustration at a positive result that may not materialize into learning. If the custom is to only reward experiments that work, or result-oriented actions, the environment ends up discouraging production and the exchange of knowledge.
This new mindset is not yet obvious to executives, who may feel intimidated by the fact that decisions are driven by the experiments themselves. The role of the boss is now to cultivate the culture and the foundations for making the entire process sustainable.
The task is made easier by accessible tools – even free of charge – and many established methodologies, such as lean product development. The real challenge is to enable and strengthen the infrastructure to carry out large-scale tests, while at the same time keeping track of collections and samples, the statistics engine and all history.
Two points are worth highlighting: the prevalence of humility over arrogance and the building of integrity and trust. It is common for experiments to clash with some belief or perception of the organization, which needs to recognize the validity of the results. In addition, preserving ethics and setting limits in relation to what is being tested brings reliability to the journey.
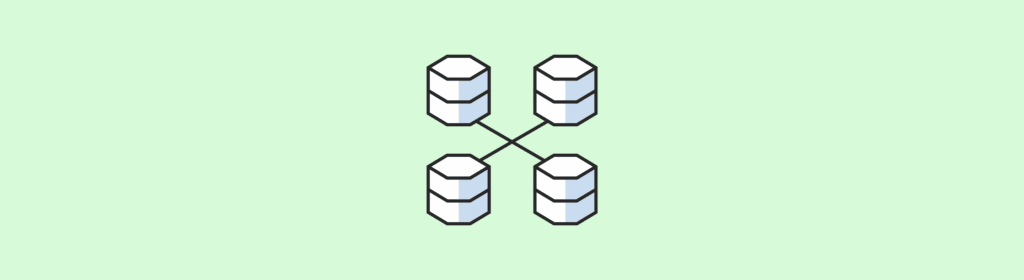
A platform to democratize data
Everything becomes concrete and flows quickly if there is a digital platform focused on data. If the intention is to validate hypotheses and leave intuition-driven management logic in the past, there needs to be a basis for these experiments. This is exemplified by the case of Joey DeBruin, who began his career in neuroscience research, worked his way up to product director and founded a company in Los Angeles, USA.
During his time at the helm of Feastly’s growth team, the platform, which connects users to chefs outside the traditional restaurant chain, experienced significant growth. Already immersed in a test and learning culture, where it was routine to run experiments, he democratized brainstorming, developing a tool to receive ideas from anyone in the company.
The invitation to think of possibilities was open – from increasing a certain indicator to improving the operation itself – but with prioritization of the hypotheses to be tested. With fully automated execution, including a control group and A/B tests to define winning and losing experiments, it was possible to evolve the experimentation process.
By providing an inclusive and open means of contributing, without giving up established rites, the gains, which were already significant due to a deep-rooted culture of experimentation, were boosted. They resulted in a tenfold increase in growth compared to the period prior to this new method. In a Reforge article, DeBruin details his rapid testing method.
We concluded that continuous improvement is fundamental to gaining maturity and a strong point when it comes to digital transformation. If there is a greater volume of executions and the capacity to run them quickly, it is possible to test more hypotheses in a shorter period and build a solid and fertile culture of experimentation – which, in turn, paves the way for innovation.
THIAGO LEITE works as partner and senior manager at EloGroup.